\(\newcommand{L}[1]{\| #1 \|}\newcommand{VL}[1]{\L{ \vec{#1} }}\newcommand{R}[1]{\operatorname{Re}\,(#1)}\newcommand{I}[1]{\operatorname{Im}\, (#1)}\)
Rotation exercise¶
>>> #: standard imports
>>> import numpy as np
>>> import matplotlib.pyplot as plt
>>> np.set_printoptions(precision=4) # print arrays to 4 DP
>>> import nibabel as nib
>>> #: gray colormap and nearest neighbor interpolation by default
>>> plt.rcParams['image.cmap'] = 'gray'
>>> plt.rcParams['image.interpolation'] = 'nearest'
Preliminaries¶
Check the you have rotations.py
in your current directory, or
elsewhere on you Python path:
>>> #: Check import of rotations code
>>> from rotations import x_rotmat, y_rotmat, z_rotmat
Your task…¶
I have taken a copy of the first volume in ds107_sub012_t1r2.nii
.
>>> #: the first volume of ds107_sub012_t1r2.nii
>>> img_4d = nib.load('ds107_sub012_t1r2.nii')
>>> data = img_4d.get_data()
>>> vol0 = data[..., 0]
Then I rotated it by:
- 0.1 radians around the x axis, then;
- 0.2 radians around the y axis, then;
- 0.3 radians around the z axis.
Here is that image for download rotated_volume.nii
.
>>> #: the resulting rotated image
>>> rotated_img = nib.load('rotated_volume.nii')
>>> rotated_vol0 = rotated_img.get_data()
>>> rotated_vol0.shape
(64, 64, 35)
Here are slices over z, y, and x, for rotated and not-rotated images
>>> #: slices on z, y, and x axis for original and rotated images
>>> fig, axes = plt.subplots(3, 2, figsize=(10, 15))
>>> axes[0, 0].imshow(vol0[:, :, 17])
<...>
>>> axes[0, 1].imshow(rotated_vol0[:, :, 17])
<...>
>>> axes[1, 0].imshow(vol0[:, 31, :])
<...>
>>> axes[1, 1].imshow(rotated_vol0[:, 31, :])
<...>
>>> axes[2, 0].imshow(vol0[31, :, :])
<...>
>>> axes[2, 1].imshow(rotated_vol0[31, :, :])
<...>
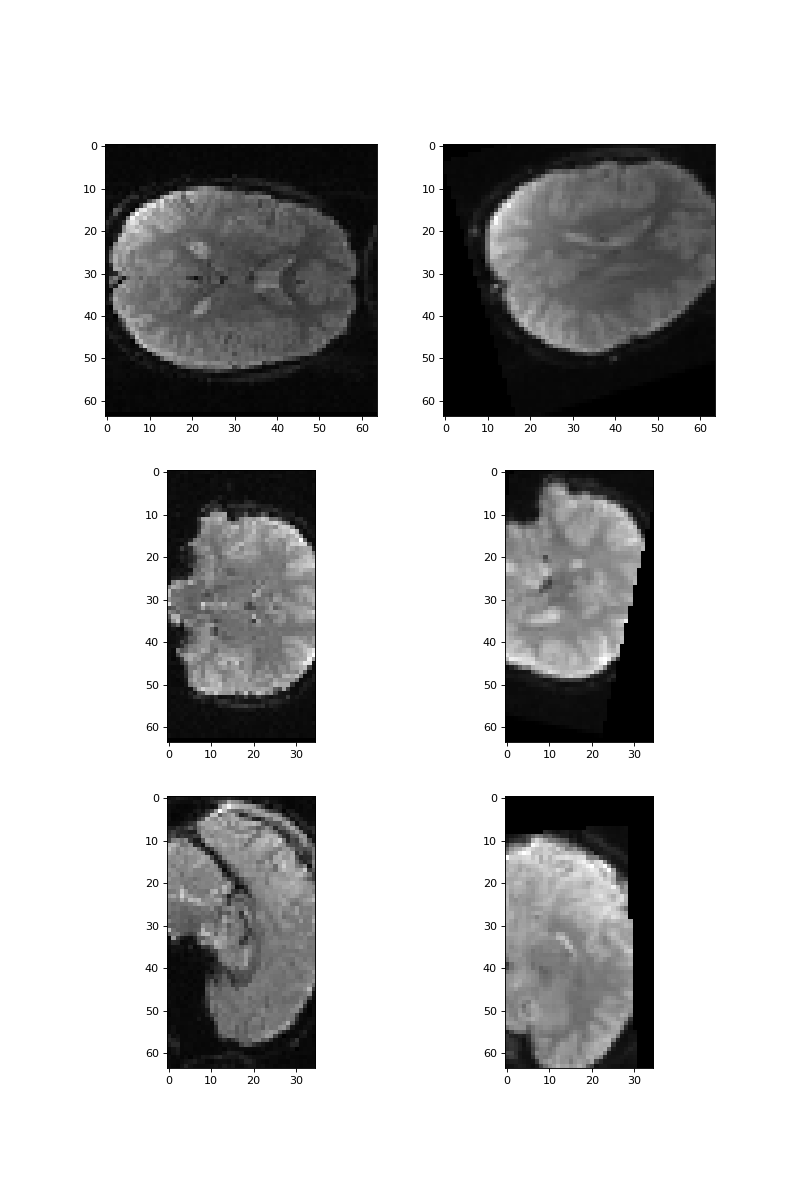
Of course, I have to be more specific by what I mean when I say that I rotated this image by:
- 0.1 radians around the x axis, then;
- 0.2 radians around the y axis, then;
- 0.3 radians around the z axis.
I am describing the mapping from coordinates in vol0
to coordinates in
rotated_vol0
.
As you now know, in order to apply this rotation, I needed to do pull
resampling, so I needed to use the mapping from coordinates in
rotated_vol0
to coordinates in vol0
. So applying this rotation
involved: rotation of -0.3 radians around z, then -0.2 radians around y then
-0.1 radians around x.
Your task, should you chose to accept it, is to make a new image, where you have undone the given rotations.
Let’s remind ourselves of what we need to do.
In order to make the new fixed image, we will need to
- Make a new empty image the same shape as the original;
- For every coordinate \((i, j, k)\) in this new empty image, we are
going to apply a transformation \(t\). This transformation will give us
the corresponding coordinate \((i', j', k')\) in the
rotated_vol0
; - We use linear resampling to estimate a voxel value \(v\) for coordinate
\((i', j', k')\) in
rotated_vol0
; - We put this voxel value \(v\) into the empty image at coordinate \((i, j, k)\).
In fact scipy.ndimage.affine_transform will do all these steps for us, but we need to supply the transformation \(t\).
So – what transformation do we need?
We are doing pull resampling - so we want the mapping from coordinates in
vol0
to coordinates in rotated_vol0
.
Your job is to make M
– a 3 by 3 matrix that applies this mapping.
See rotation in 2d and Rotations and rotation matrices.
M
is the matrix that scipy.ndimage.affine_transform
will use to get
the transformation between coordinates in the new empty image and the
corresponding coordinates in the rotated image.
>>> #- Make a 3 by 3 transformation matrix that applies this sequence
>>> #- * 0.1 radians around the x axis, then
>>> #- * 0.2 radians around the y axis, then
>>> #- * 0.3 radians around the z axis.
>>> M = z_rotmat(0.3).dot(y_rotmat(0.2)).dot(x_rotmat(0.1))
>>> M
array([[ 0.9363, -0.2751, 0.2184],
[ 0.2896, 0.9564, -0.037 ],
[-0.1987, 0.0978, 0.9752]])
Use scipy.ndimage.affine_transform
to create the new image and use the
transform to sample from the rotated image:
>>> #- Use affine_transform to resample from `rotated_vol0` using the transform.
>>> #- Get the result into a variable `derotated_vol0`
>>> from scipy.ndimage import affine_transform
>>> derotated_vol0 = affine_transform(rotated_vol0, M)
>>> derotated_vol0.shape
(64, 64, 35)
>>> #- plot slices from the image to see if you got the right transformation.
>>> fig, axes = plt.subplots(3, 2, figsize=(10, 15))
>>> axes[0, 0].imshow(vol0[:, :, 17])
<...>
>>> axes[0, 1].imshow(derotated_vol0[:, :, 17])
<...>
>>> axes[1, 0].imshow(vol0[:, 31, :])
<...>
>>> axes[1, 1].imshow(derotated_vol0[:, 31, :])
<...>
>>> axes[2, 0].imshow(vol0[31, :, :])
<...>
>>> axes[2, 1].imshow(derotated_vol0[31, :, :])
<...>
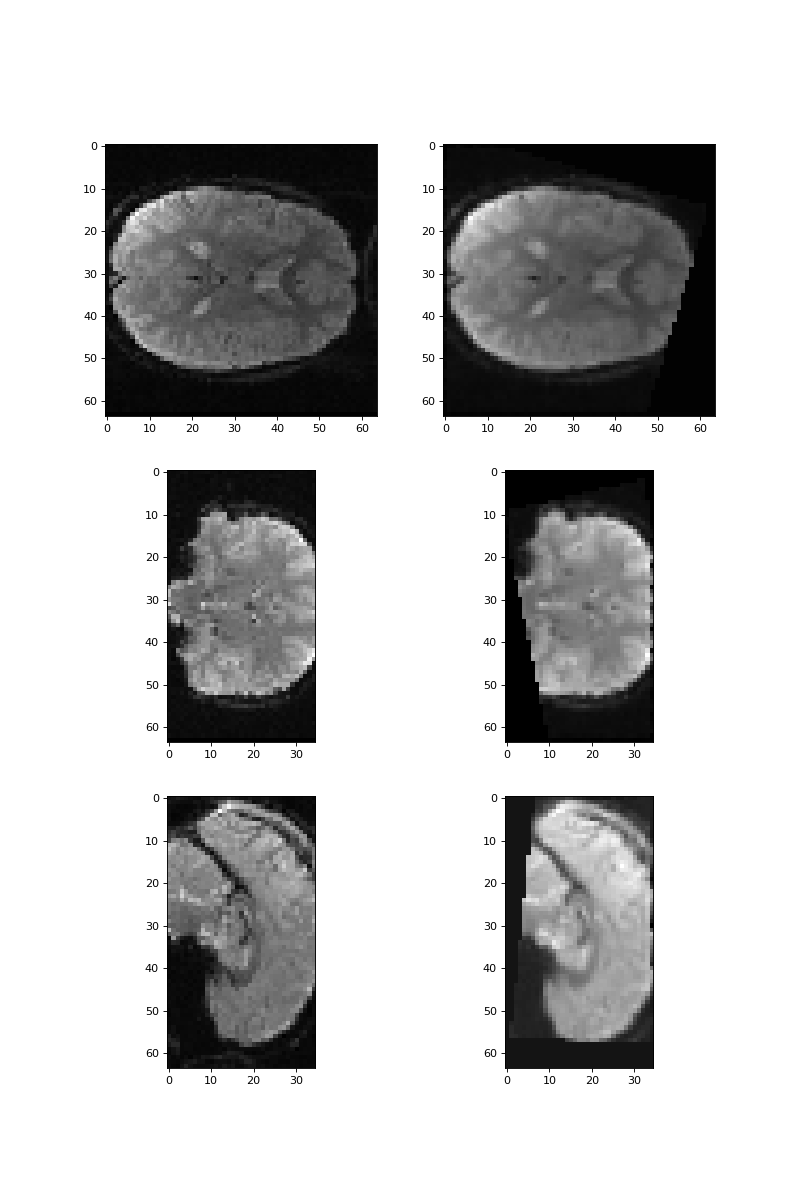