\(\newcommand{L}[1]{\| #1 \|}\newcommand{VL}[1]{\L{ \vec{#1} }}\newcommand{R}[1]{\operatorname{Re}\,(#1)}\newcommand{I}[1]{\operatorname{Im}\, (#1)}\)
Anatomical solution¶
>>> #- Our usual imports
>>> import numpy as np # the Python array package
>>> import matplotlib.pyplot as plt # the Python plotting package
Now we will work with a 3D brain image.
Like the camera image in Cameraman solution, the pixel data for the 3D
image is in a text file called anatomical.txt
. Download
anatomical.txt
to your working directory.
I happen to know this image has length 32 on the third dimension, but I don’t know what the size of the first two dimensions are.
So, I know the image is of shape (I
, J
, 32), but I don’t know
what I
and J
are.
Here are the first four lines of anatomical.txt
.
0.0000
0.0000
53.0000
43.0000
The data is in the same floating point text format as the camera picture pixel data.
Read all the lines of the file into a list of float values, as before.
>>> #- Read file into list of float values
>>> pixel_values = []
>>> for line in open('anatomical.txt', 'r'):
... pixel_values.append(float(line))
How many pixel values does this file contain?
>>> #- How many pixel values?
>>> len(pixel_values)
848640
When I have my image array correctly shaped, then, if I take a slice over the third dimension:
slice_on_third = image_array[:, :, 0]
slice_on_third
will be shape (I, J)
(the size of the first two
dimensions).
How many pixel values does a slice in the third dimension contain - given that
we know the third dimension is length 32? Put another way, what is the value
for I * J
?
>>> #- Find the size of a slice over the third dimension
>>> # P = ?
>>> P = len(pixel_values) / 32
>>> P
26520.0
Call P
the number of values per slice on the third dimension (so P == I
* J
where we don’t yet know I
or J
).
Is this slice over the third dimension square (does I == J
)?
We need to find the values for I
and J
.
Find candidates for I
by using the modulus operator (%
) to find a few
numbers between 120 and 200 that divide exactly into the slice size P
.
Hint: the first value will be 120.
>>> #- Find candidates for I
>>> candidates = []
>>> for i in range(120, 201):
... if P % i == 0:
... candidates.append(i)
>>> candidates
[120, 130, 136, 156, 170, 195]
These numbers are candidates for I
- the first number in the pair (I,
J)
. We now need to find the corresponding J
for each candidate for
I
.
Use the integer division operator (//
) to get a list of pairs of numbers
I
and J
such that I * J == P
. Hint: the first pair of I, J
is
(120, 221).
>>> #- Find candidate pairs for I, J
>>> pairs = []
>>> for candidate in candidates:
... pair = [candidate, P // candidate]
... pairs.append(pair)
>>> pairs
[[120, 221.0], [130, 204.0], [136, 195.0], [156, 170.0], [170, 156.0], [195, 136.0]]
The full image shape will be three values, with one of these (I, J)
pairs
followed by 32. For example, the correct shape might be (120, 221 , 32) (it
isn’t!). Try reshaping the pixel data with a few of the (I, J, 32)
candidates to see which one is likely to be right. You might want to plot a
slice over the third dimension to see how it looks.
>>> #- Try reshaping using some candidate pairs
>>> pixel_array = np.array(pixel_values)
>>> try1 = np.reshape(pixel_array, (130, 204, 32))
>>> plt.imshow(try1[:, :, 15]) # A middle slice in the third dimension
<...>
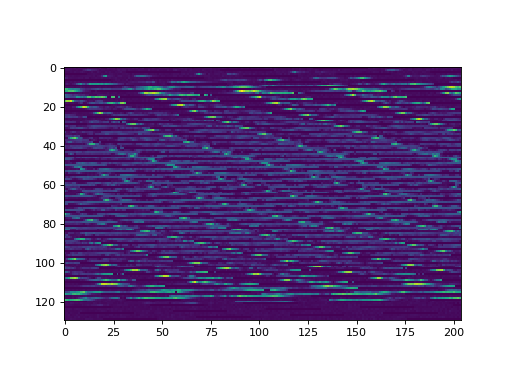
>>> try2 = np.reshape(pixel_array, (195, 136, 32))
>>> plt.imshow(try2[:, :, 15]) # Same slice
<...>
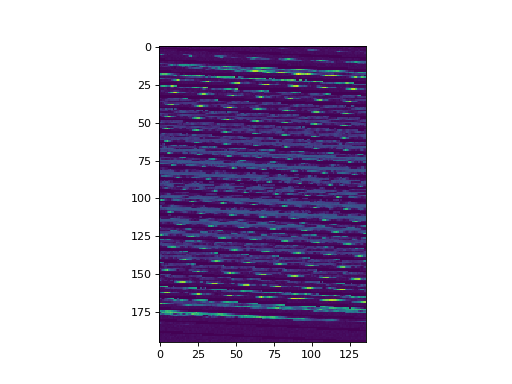
>>> try3 = np.reshape(pixel_array, (170, 156, 32))
>>> plt.imshow(try3[:, :, 15])
<...>
>>> # The last one looks good, so final shape is:
>>> try3.shape
(170, 156, 32)
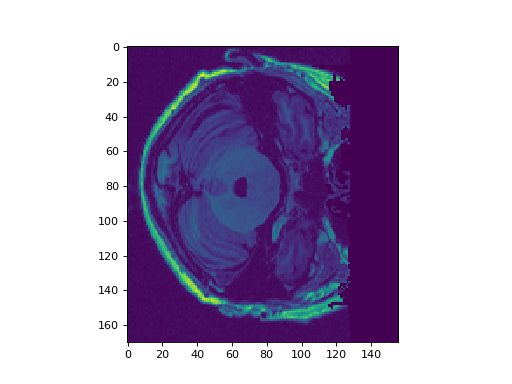