\(\newcommand{L}[1]{\| #1 \|}\newcommand{VL}[1]{\L{ \vec{#1} }}\newcommand{R}[1]{\operatorname{Re}\,(#1)}\newcommand{I}[1]{\operatorname{Im}\, (#1)}\)
Applying deformations exercise¶
- For code template see:
applying_deformations_code.py
; - For solution see: Applying deformations exercise.
Requirements:
- Removing length 1 axes with numpy.squeeze;
- numpy.tranpose for swapping axes;
- Resampling with scipy.ndimage;
- The nibabel.affines module;
- General resampling between images with scipy.ndimage.map_coordinates.
>>> #: standard imports
>>> import numpy as np
>>> import matplotlib.pyplot as plt
>>> # print arrays to 4 decimal places
>>> np.set_printoptions(precision=4, suppress=True)
>>> import numpy.linalg as npl
>>> import nibabel as nib
>>> #: gray colormap and nearest neighbor interpolation by default
>>> plt.rcParams['image.cmap'] = 'gray'
>>> plt.rcParams['image.interpolation'] = 'nearest'
Applying a deformation field¶
We should have already calculated the deformation field for the image
ds107_sub012_highres.nii
.
We did this using the SPM12 Normalise: Estimate
option from the GUI.
This should have left an image called y_ds107_sub012_highres.nii
in
your working directory.
If not:
- Ask for help;
- If you get stuck, you can download the image from
y_ds107_sub012_highres.nii
.
We make an image object for this deformations image by loading with nibabel.
>>> #: load y_ds107_sub012_highres.nii with nibabel
>>> # get the image array data
>>> deformations_img = nib.load('y_ds107_sub012_highres.nii')
>>> deformations_data = deformations_img.get_data()
>>> deformations_data.shape
(121, 145, 121, 1, 3)
We are going to work out how to apply this deformations image to reslice our
original image ds107_sub012_highres.nii
.
Oddly - this is a 5 dimensional image, where the 4th dimension is length 1.
The length-1 4th dimension is an artefact of the NIfTI image format – so let’s get rid of this dimension with np.squeeze:
>>> #: remove length-1 4th dimension from deformation data
>>> deformations_data = np.squeeze(deformations_data)
>>> deformations_data.shape
(121, 145, 121, 3)
The data is now a 4-dimensional image, containing 3 volumes. These volumes are:
- x coordinates;
- y coordinates;
- z coordinates
respectively.
Put another way, the vector deformations_data[i, j, k, :]
gives the (x, y,
z) coordinates for the voxel [i, j, k]
. More on this later.
If you were looking carefully at the SPM interface, SPM has calculated the
distortions necessary to go from a template of tissue probability maps
(called TPM.nii
) to the ds107_sub012_highres.nii
image.
We can get the original 3D shape and affine of TPM.nii
because SPM stored
them in y_ds107_sub012_highres.nii
:
>>> #: get original TPM.nii 3D shape and affine
>>> tpm_shape = deformations_data.shape[:3]
>>> tpm_affine = deformations_img.affine
>>> tpm_affine
array([[ -1.5, 0. , 0. , 90. ],
[ 0. , 1.5, 0. , -126. ],
[ 0. , 0. , 1.5, -72. ],
[ 0. , 0. , 0. , 1. ]])
First we look at the images before the normalization has been applied.
To do that, we will make a new copy of the MNI template, with the same shape as the TPM image.
The MNI template image we will use is
mni_icbm152_t1_tal_nlin_asym_09a.nii
.
>>> #: load the template image we will resample from
>>> template_img = nib.load('mni_icbm152_t1_tal_nlin_asym_09a.nii')
>>> template_data = template_img.get_data()
>>> template_data.shape
(197, 233, 189)
Now we need the mapping from voxels in TPM.nii
to voxels in
mni_icbm152_t1_tal_nlin_asym_09a.nii
.
We can break this down into two transforms:
- from voxels in
TPM.nii
to (x, y, z) mm; - from (x, y, z) mm to voxels in
mni_icbm152_t1_tal_nlin_asym_09a.nii
.
We have these transforms already. The full transform is:
>>> #: voxels in TPM.nii to voxels in mni_icbm152_t1_tal_nlin_asym_09a.nii
>>> # Matrix multiplication is right to left
>>> vox2vox = npl.inv(template_img.affine).dot(tpm_affine)
>>> vox2vox
array([[ -1.5, 0. , 0. , 188. ],
[ 0. , 1.5, 0. , 8. ],
[ 0. , 0. , 1.5, 0. ],
[ 0. , 0. , 0. , 1. ]])
We break this down into the 3 x 3 mat
and length 3 vec
components:
>>> #: to mat and vec
>>> mat, vec = nib.affines.to_matvec(vox2vox)
>>> mat
array([[-1.5, 0. , 0. ],
[ 0. , 1.5, 0. ],
[ 0. , 0. , 1.5]])
>>> vec
array([ 188., 8., 0.])
Then we resample from the MNI template, into the voxel grid of the
TPM.nii
:
>>> #: resample MNI template onto TPM grid
>>> from scipy.ndimage import affine_transform
>>> template_into_tpm = affine_transform(template_data, mat, vec,
... output_shape=tpm_shape)
>>> template_into_tpm.shape
(121, 145, 121)
Here is the new version of the template image:
>>> #: plot the template image resampled onto the TPM grid
>>> plt.imshow(template_into_tpm[:, :, 60])
<...>
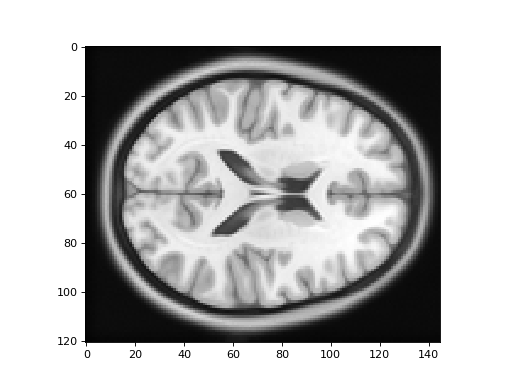
Now, what to do with with the SPM distortion field in deformations_data
?
By checking in the SPM source code [1], it is possible to
work out that deformations_data
contains, for every voxel in TPM, the
corresponding mm coordinate in the mm space of the subject image.
That is, deformations_data[i, j, k]
is a length 3 vector [x, y, z]
where [x, y, z]
are the mm coordinates of voxel [i, j, k]
when mapped
into millimeters for the subject image.
Here is the subject data, and the image, which contains the affine:
>>> #: load the subject data that we will resample from
>>> subject_img = nib.load('ds107_sub012_highres.nii')
>>> subject_data = subject_img.get_data()
>>> subject_data.shape
(256, 208, 192)
With this information, and with the scipy.ndimage.map_coordinates
function, you should be able to:
- get the mapping from voxels in TPM to voxels in the subject image and;
- resample the subject image into the grid of the TPM image using this mapping.
Hint: remember that map_coordinates expects the
3-length coordinate dimension to be first, but deformations_data
– at
the moment – has the 3-length coordinate dimension last.
>>> #- * get mapping from voxels in TPM to voxels in the subject image;
>>> #- * resample the subject image into the grid of the TPM image using
>>> #- this mapping.
Show an example slice from the template resampled into the TPM voxel grid, and the subject resampled into the TPM voxel grid:
>>> #- show an example slice from the resampled template and resampled
>>> #- subject
Footnotes
[1] | It’s not very easy to work through the SPM12 source
code for this, but if you want to check for yourself, you’ll find that the
SPM batch interface for “Normalise: Write” is in config/spm_run_norm.m.
The batch interface calls the sub-function norm_write.
norm_write collects the NIfTI file with the y_ prefix that contains
the deformations, if the user did not specify the file, and calls the
spm_deformations function. This function in turn calls down into the
pull_def
sub-function, in which SPM calculates the matrix Y , which is the (I, J,
K, 3) image data from the deformations NIfTI file, left multiplied by the
mm to voxel mapping for the image being resampled. pull_def then
resamples from the image to which we are applying “Normalize: Write”, using
the Y array as voxel coordinates. Therefore the y_ -prefixed
deformation field image contains the coodinates mapping from voxels in the
template to millimeters in the image that was registered. |